Data Justice and Singapore’s Smart Nation
Silence, Noises and Semantics
Team Members
Linnet Taylor,
PhD., TILT (Tilburg University)
Shazade Jameson, MSc., TILT (Tilburg University)
Josh Bullock,
PhD. (Kingston University)
Quynh Tu Hoang (University of Amsterdam)
Jeroen de Vos, facilitator (University of Amsterdam)
Maarten van Gestel (University of Amsterdam)
Timo Nijssen (University of Amsterdam)
Olivia Dziwak (York University)
Kristoffer Rekve (Aalborg University)
Yoren Lausberg (University of Amsterdam)
Stefany Winona Santosa (University of Amsterdam)
Wen Yang (University of Amsterdam)
Giovanni Zenga (University of Milan)
Contents
Summary of Key Findings
We aimed to map the networks and key concepts involved in Singapore’s ‘Smart Nation’ initiative from the perspective of the Singaporean authorities, and to map and analyse the popular response to datafication.
We found that the authorities’ narrative is clear and replicated across multiple online sources. It is authored by a mixture of government and commercial actors and has strong resonance with international discourse on smart cities. It is principally hosted via Facebook and websites belonging to the government and its partners, and there is little engagement (regarding response/re-sharing) visible online from citizens.
We were able to map the official discourse quite quickly, but a widespread/critical counter-narrative was harder to find, draw out and analyse. We found that the visible critical response to the smart nation initiative revolves principally around functionality and efficiency (‘this does not work as promised’) and that there are no clearly visible public threads of discourse around rights or surveillance in relation to data. We found concerns with datafication mainly on local news sites and Reddit.
This analysis has mainly been used to help us to identify gaps and silences on the side of citizens. The social media sources with the highest penetration in Singapore carry the government narrative almost exclusively. Those with lower penetration have some responses from citizens, but in general, the public-facing component of the smart nation initiative is governmental.
Critical voices in relation to Singapore’s datafication are largely unavailable to remotely conducted digital methods. We conclude from our investigation that it is worth using digital methods to analyse the government narrative on datafication, but that researchers hoping to identify the alternative narratives should initially do so through ethnographic fieldwork and through that generate questions that are more amenable to digital methods.
1. Introduction
Imagine your morning routine in a fully connected world. You wake up when the
SmartMeter opens the blinds, adjusts the temperature and maybe plays your favourite Spotify tunes. As you go to work, the Municipality app warns you of issues on the Metro and automatically calls a driverless Uber, which you can pay for with your contactless wristwatch. You get a real-time notification from the robot who is caring for your parents that all is well, and you’re ready to start your day. This vision is not far from the current reality in Singapore.
Singapore is one of the world’s most highly connected countries and ranked by the World Economic Forum as the best prepared for the digital economy. It is also one of the most surveilled countries and ‘smart cities’ in the world. This year, the Government of Singapore launched their ‘Smart Nation’ initiative, to use advances in digital technology to support society and the development of the city across all major sectors. It includes, for instance, a nationally-integrated sensor network, cashless and contactless payment systems for autonomous buses, AI for healthcare, troves of open data, and vast investment to drive tech innovation. Nowhere else is datafication being implemented as an urban intervention to such a scale.
Yet the city is also a laboratory for surveillance technologies, and for this reason is one focus of our new ERC
DataJustice project. We are interested to know how people are perceiving the benefits and risks of living in a city which is continuously monitored, and how their needs and opinions are being expressed and – possibly – taken into account by the state.
We want to map the discussion communities around datafication as an intervention into urban life in Singapore. By doing this, we would like to identify the narratives and debates that accompany datafication: what are people excited about? What are they concerned about? Do people discuss privacy concerns, and if so, in what context?
2. Initial Data Sets
Initial data sets included:
• ‘smart_cities’ bin on TCAT4, this is a set of over 5M tweets scraped with the query: ['ciudad inteligente', 'ciudades inteligente', 'slimme stad', 'smart cities', 'smart city', ciudadesinteligente, ciudadinteligente, slimmestad, smartcities, smartcity]
• Two Reddit bins accessed through
BigQuery - provided by Jason Baumgartner and Felipe Hoffa. These bins contain all content created on Reddit, scraped and uploaded month-by-month
• List of key (critical) terms regarding ‘smart nation’ initiative, generated by online research
We then created several other data sets, including texts scraped from Facebook, Reddit, and the wider Internet, to be described below.
3. Research Questions
1. What are the central positive institutional, policy and popular narratives around new data technologies in Singapore?
2. What alternative narratives are emerging in response, and what principles or objectives do they focus on?
4. Methodology
As exploratory research, the general approach of the project was iterative, running several small pilots in parallel to try out methods, reflecting on findings, and then pushing the findings in new directions.
We conducted a cross-platform analysis of public commentary sourced from
• Facebook (Netvizz &
CrowdTangle),
• News articles (Google scraper and Cortext combined),
• Twitter (DMI-TCAT and
DiscoverText),
• Reddit (Google scraper /
BigQuery)
Query design:
• ["Smart nation"],
• ["singapore" OR "singaporean" OR "SG" AND "smart city" OR "smart nation"],
• ["gahmen" AND "smart city" OR "smart nation"].
Facebook Firstly, with the use of Netvizz we identified the principal actors in the Smart Nation Singapore issue space by crawling the Facebook page like network from the seed of the Facebook page “Smart Nation Singapore" (crawl depth: 2). The Facebook Page Like Network helped us to make informed choices of the main pages for data extraction. We scraped data of Facebook pages of two media companies (The Straits Times and Channel News Asia), two political actors (Lee Hsien Loong and Vivian Balakrishnan) and two governmental organisations (
GovTech and Smart Nation Singapore) by Netvitzz. From these data, we filtered the relevant posts in relation to the Singapore’s Smart Nation Initiative by searching and matching keywords. The comments made on the filtered posts are subsequently extracted for further textual analysis.
We also explored Facebook using
CrowdTangle in two different ways. One way was to manually identify individual news stories and other web pages and then use the
CrowdTangle Chrome Extension to see on what Facebook pages and Twitter profiles it was posted and what engagement it got. This was interesting to look at the relevance of individual stories but didn’t prove to be useful for gathering data.
The other way we employed
CrowdTangle was by using the apps.crowdtangle.com website where we set up two dashboards: one with the Facebook pages of the major news brands in Singapore and another dashboard with the Facebook pages of politicians Lee Hsien Loong (the prime minister) and Vivian Balakrishnan (the minister in charge of the Smart Nation project). These dashboards were used to search for posts with terms related to smart nation (e.g. ‘cashless’). The output of these searches consisted of a .csv file with the full post text, web pages linked to, the number of likes and other reactions, shares and the number of comments (but not the content of these comments). It is also possible to see how well a post was performing (although
CrowdTangle does not define what performance is: engagement or reach) relative to the average of the page. The downside of using
CrowdTangle is that it only allows searches within 4-month timeframes and that it only shows results that are either over- or underperforming, not both.
Google We used Google Scraper to generate a list of the top 100 websites associated with key [smart nation] terms and analysed the proportion of government/business/academic actors involved. We also used Google Scraper to generate a list of websites associated with the key critical terms we had found through qualitative analysis. We then used
DiscoverText to generate word clouds based on the frequency of specific terms. We further input scraped results (from government websites and facebook) to topic modelling tools MALLET and Google Topic Modelling (using both asymmetrical and symmetrical topic modelling), running several iterations and creating a custom stopword lists to clean the dataset of URLs, producing a list of topics characterising the government narrative.
Twitter We approached Twitter through a two-pronged approach: looking at older tweets (beginning in 2015) in the ‘smart cities’ bin collected in the DMI-TCAT tool, and at live tweets through
DiscoverText, which collected tweets using the search terms [“smartnation” OR “smart nation”]. In both datasets, there was a large amount of noise, which consisted of bot-generated tweets, irrelevant advertising, and false-hits, for example pertaining to the Italian “Smart Nation” initiative (
https://smartnation.it/). For example, of the ~300 tweets collected through
DiscoverText, roughly 60% were deemed “useful” in our coding process, meaning they referred, either directly or indirectly, to the Singaporean Smart Nation agenda. These “useful” tweets were an early indication of the dominance of the government narrative, containing predominantly pro-Smart Nation articles or advertisements. Overviews of term frequency in this corpus of tweets supported this qualitative impression, with terms like “talent,” “society,” and “future” standing out most prominently.
We scraped from the TCAT dataset data related to [“singapore”] and [“smart nation OR smartnation”] keyword from 2015-07-07 to 2018-01-08, in two different queries that respectively resulted in 7400 and 34000 tweets. We look at most used co-hashtags trying to map the dominant narrative and find traces of a public counter-narrative. The result was a raw list of co-hashtags ordered by absolute frequency.
In order to contrast the topics found in the government narrative, we also wanted to investigate to which other topics the term “smart nation” was being used in a broader, global media picture, to see if any other topics would appear as relevant. We did a small project in which we sampled 10 articles from a list of 15 English-Singaporean news outlets found online. For all of these sites, a site-specific Google query was performed, yielding the top articles for the term [“smart nation”], after which the full text from these articles was placed in a spreadsheet. As several sites did not yield a full 10 articles, and still others were inaccessible, the sample size ended up smaller than hoped, being made up of little more than 80 articles. Nonetheless, the sample was put into Cortext. Here, a terms list of 200 words were extracted, which helped produce a discourse network map.
Reddit We used Google Big Query to explore the /r/singapore subreddit searching terms related to the Smart Nation project. We create a dataset with all comments related to “smart nation” and then added to our query other keywords to map the public conversation, based on the language of the ‘Initiatives’ section of the smartnation.sg website to better see if we could map the response on Reddit to these government initiatives. We also searched for ‘Gahmen’ (Singaporean English -Singlish- word for government) and create a list of URLs for the relevant discussions. We converted the outcome of our queries to a .txt file to feed to the Topic Modelling Tool and run it with 2000 iterations to obtain 15 topics. The result is a list of topics that reflect in broad strokes the language used to discuss the Smart Nation initiative, which in turn can be compared to the language used by (semi) government actors that push the Smart Nation agenda.
5. Findings
Twitter co-hashtags maps

Figure 1. Most used co-hashtag frequency map with ego-network Gephi visualization for “smart nation” resulting from “smart nation” query in “smartcities” TCAT dataset.
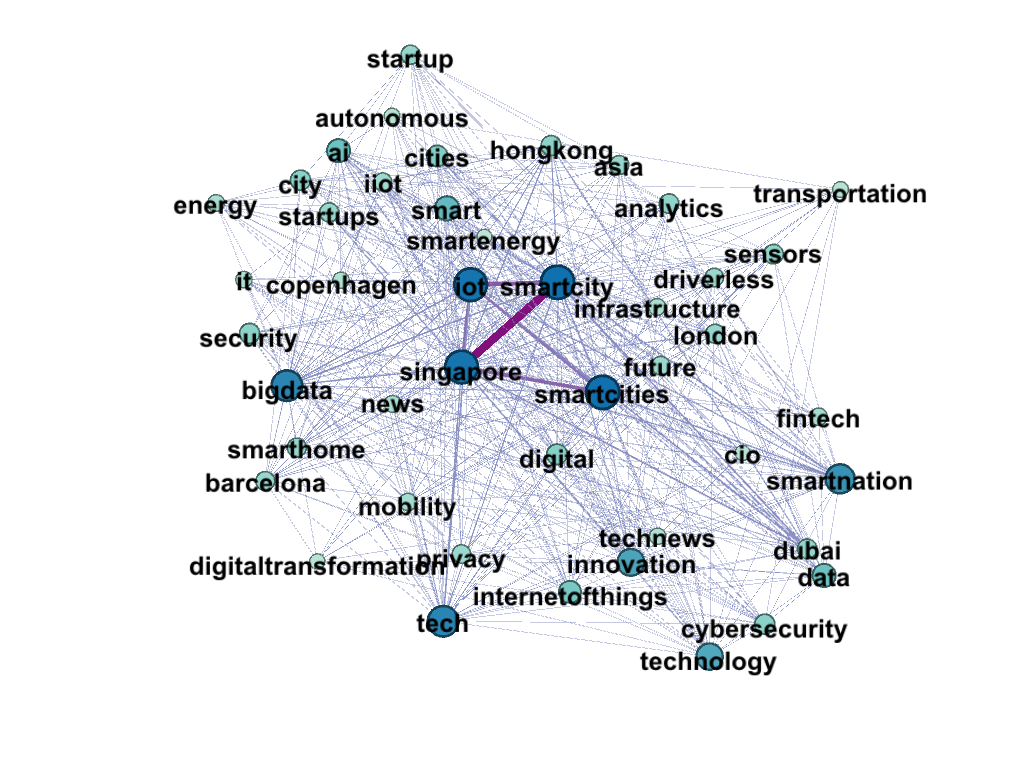
Figure 2. Most used co-hashtag frequency map with ego-network Gephi visualization for “singapore” resulted from “singapore” query in “smartcities” TCAT dataset.
What the network visualization for both our queries of most-used co-hashtags (“singapore” and “smart nation”) shows is coherent with the government narrative present on other media:
• smart nation narrative is highly present and seems to form a coherent ecosystem of information;
• the hashtags reflect a corporate agenda (
IoT /sensors, ai, innovation, smart homes, big data, find tech;
• alternative narratives are not present or not publicly recognizable
Facebook Networks
We used the Facebook like network mapping with Netvizz to identify the most dominant actors that are involved in the Singapore’s Smart Nation (see Figure 3). We identified numerous political actors, government agencies, and news media outlets, with the most prominent clusters being the public actors at the forefront of the Smart Nation initiative.
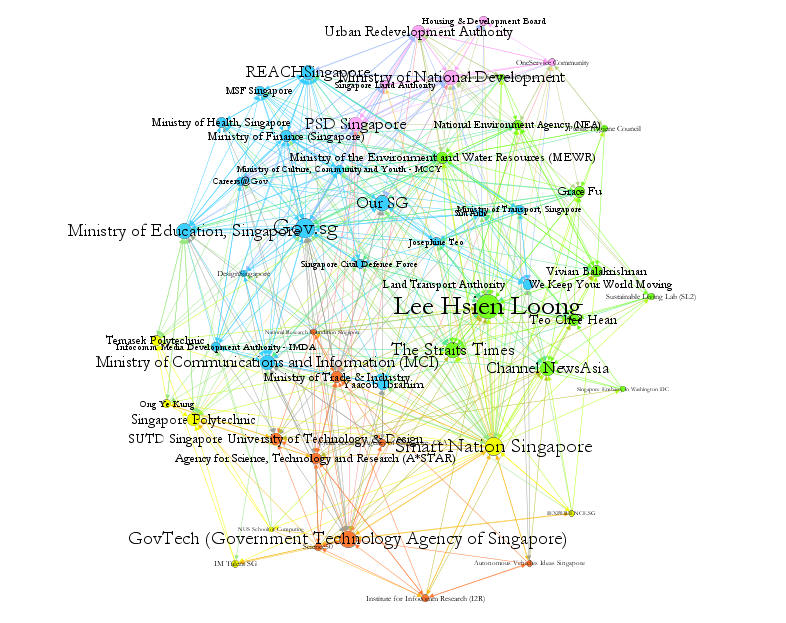
Figure 3. Smart Nation Singapore page like network (Netvizz). Color represents statistical communities.
As seen from Figure 3, the most influential actor in the Smart Nation initiative, with the largest green node, is Singaporean Prime Minister Lee Hsien Loong. Vivian Balakrishnan, the Minister-in-charge of the Smart Nation Programme Office is also a dominant actor, along with major ministries such as
GovTech and the Ministry of Communications. The major media sites reporting the most on the initiative include Channel News Asia and The Straits Times. The mapping also shows that it is a relatively closed and homogeneous network, much like the Twitter hashtag network.

Figure 4. Word Cloud of the 100 most used phrases in Singapore government's Facebook posts related to Smart Nation Initiatives.
Posts from the most prominent government actors that mention smart nation are predominantly promotional, with little alternative narratives or responses offered in the comment, and it is thus not easy to tell a neutral story about Singaporeans’ view toward the smart nation initiative only from politicians’ Facebook pages. The same is true for the pages of official page of “Smart Nation Singapore” and other governmental organizations.
Topic Modelling government response

Figure 5: Comparison of two topic models; one from Reddit discussions and one from Government Facebook posts on the Smart Nation
By topic modelling on two sets of data, one being the government’s posts from Facebook and the web scraper on the Smart Nation, and the other being the discussions on Reddit, we were able to see what substantive issues people were most concerned about, and which substantive issues the initiative is acting upon. The two topic models each produced 15 topics; though as the government facebook data set was relatively small there were fewer topics which could be clearly defined. Seeing the two lists of topics (Figure 5), there is clearly a correlation between the different topics, such as digital employment, housing, coding, education and the elderly. There were some differences, such as where government topics were more concerned with global competitiveness and positioning the city. Interestingly, both sets of topics had one cluster which was largely just verbs and served a function within their dataset. For Reddit, the verbs and verb phrases were about discussing, reacting to another post, offering an opinion. For the government, the verbs and phrases were clearly call-to-actions at the end of Facebook posts, asking for engagement (e.g. ‘make’, ‘share’, ‘read’).
Facebook comment responses

Figure 6. Word cloud showing concerns shared in Facebook comments.
While the government naturally promotes the Smart Nation program as “innovative," “cutting-edge," “digital revolution," and “cool tech,” on social media some people raised concerns about the project. These concerns were mostly around achievability, and about whether initiatives would effectively address the problems.
Figure 6 draws out the most common concern shared in Facebook comments. People responded most on the issue that seniors cannot keep up the pace the of sociotechnological changes; commenters were concerned about elderly being locked out of recent digital innovations. Another issue that was raised by citizens concerned the risks associated with the ‘cashless’ turn. Prime Minister Lee Hsien Loong claimed that Singapore should learn from China’s experience, where in many cities cash and even debit or credit cards have become obsolete. However, the Singaporeans who commented seem to worry that a cashless society may run into security problems, such as hackers breaking into phones and stealing e-money or making fraudulent transactions. Other prominent concerns included the loss of jobs and the effectiveness and efficiency of government initiatives.
News article clusters

Figure 7. Broader media narratives around Smart Nation; Coretext network diagram scraped from 15 news media websites
In mapping the popular media articles mentioning Smart Nation (see Figure 7), the leading clusters were about ‘digital government’, ‘scanning qr codes’, and ‘lamp posts’. Diving further into the data itself, these were related to recent speeches by the Prime Minister on smart data and the smart nation initiative, focusing mainly on the e-payment initiative.
The two outlier topics are relatively interesting; the first being about cybersecurity and cyberattacks, which was a definite outlier and not connected to the main Smart Nation discussions. The second outlier could be considered noise because it discusses ‘vulcan posts’ and ‘potato princes’.
6. Discussion
The central narrative we found from the Smart Nation program was a coherent, relatively homogenous message, actively promoting the potential of digital and smart city technology to increase efficiency and harmony in the city-state. This narrative played out in different ways on different platforms; Facebook sought to engage people, and indeed there were many comments, Twitter was more of a promotional echo chamber and reflected more of the global, private sector marketing hashtags, and the results from scraping the web and news sites were more responsive to events, such as particular speeches. These different flavours and dynamics could prove to be one avenue to explore in future research, in how the different cultural contexts around each platform shape how discourses and dialogues are shaped.
The alternative narrative we found emerging in response was the widespread, popular experience of datafication in the city, and centered largely around concerns of efficiency, usability (particularly for the elderly), (cyber) security and cost-effectiveness. We did not find other dissenting voices or networks online, suggesting that they may be effectively hidden from (digital) view. The recurring themes people discussed were reflected across platforms, which reinforces the suggestion that these are people’s legitimate concerns. We did find some international comment on the Smart Nation initiative, particularly regarding its scale. Interestingly, despite the governments’ push for open data as being a foundation of the smart city data strategy, we did not find a voice of the global open data community discussing the potential of the Smart Nation project as a new sandbox.
While analyzing the comments made by Singaporeans, it is noticeable that citizens sometimes express their criticism in the form of sarcasm, for example: ‘Look at this SMART solution! #laughdieme’. Although this form of expression sometimes complicates our research in deciding whether it is positive or negative towards Smart Nation initiatives, it proves that a qualitative analysis is vital to understand narratives as a computational research method is not able to make such distinction yet.
Which brings us to a short reflection on methods. Particular methods only fit specific questions, and internet research can be inherently messy. In that sense, as a form of data analysis, it is most useful with a large sample size, such as national or international conversations that the Internet facilitates. While Singapore is a nation, it is also a small nation, and in terms of population size is relatively local. There are four dominant languages in Singapore, with English being only one of them; and since we were limiting ourselves to English only, this introduces a language bias to our research. As a result, the types of local level analysis that this project attempted to undertake was repeatedly methodologically hampered by a small sample size, and thus the methods were not always suited.
Therefore, the use of, for instance, Topic Modeling as a method, should be seen in light of exploring both the content and the method itself. Topic modelling is very suitable for exploring large bodies of structured and well-embedded data, and thereby in extension, the use of Topic Modelling in context of series of more fragmented scrapes of individual posts, pages and comments was a bit of a gamble. Nevertheless, the results gave us new insights mostly into the vernacular used in the government-backed agenda in comparison to the way people would discuss the issues in question.
The significant noise in the data re-emphasized the need for cleaning the data. Creating and updating the stopword list in topic modelling was instructive as it must be content- and context-specific; for example, we found that we have to remove ‘infrastructural text’ like ‘sg’ and ‘http’ as stopwords, amongst others, as other URLs were being pulled as links. The process of data cleaning is then a conversation with the data itself. We experimented with using machine learning methods to code the dataset for relevance using
DiscoverText, but the sarcasm and difficulties of sentiment analysis were not possible and were not giving coherent results in a short space of time.
Ultimately, we drew extensively on the use of network diagrams and wordles, but these can only be but a first exploratory foray into the work. They are useful as an initial step into researching the topic, which would need to be complemented by other methods. Drawing some inferences, such as the comparison between topic models, is largely correlational, but could be an interesting future avenue. In general, the lesson learned is to follow the themes and questions not the tools alone.
7. Conclusions
We found that digital methods are an ideal way to initially research Singapore’s smart nation project (and smart city projects in general) since such projects have a strong public-facing digital campaign for support and popular recognition. To some extent this worked, as there were responses online, but the majority of posts were within the government’s network of politicians, technical ministries and contracting academic institutions. There was no widespread dissent discourse visible in reaction to the increasing datafication, voicing civil rights or freedom of speech in general.
Methodologically, we found that small-N discussions of the sort found in a small state in response to a government initiative are not amenable to some digital methods (especially topic modelling). We also found that the best way to research contentious speech in such contexts is to generate keywords through qualitative analysis (for example, issues such as cybersecurity pop out from the local news). We therefore conclude that while it is possible to sketch the dimensions, characteristics and networks relating to the government’s position adequately online, it is not realistic to find the whole range of responses using the same methods. Generation of the right terms through qualitative analysis might help researchers refine research questions and methods specific to those counternarratives and, in turn, to conduct effective analyses.
8. References
Crates, E. (2017, May 2). ‘It’s important no one gets left behind’: Singapore’s government data strategy. Retrieved 23 January 2018, from
http://www.theguardian.com/public-leaders-network/2017/may/02/singapore-government-data-strategy-jacqueline-poh
Johnson, J. A. (2014). From open data to information justice.
Ethics and Information Technology,
16(4), 263–274.
Melville, S., Eccles, K., & Yasseri, T. (2017). Semantic Map of Sexism: Topic Modelling of Everyday Sexism Project Entries.
ArXiv Preprint ArXiv:1711.09074.
Privacy International (2017). Smart Cities: Utopian Vision, Dystopian Reality.
Privacy International.
Shaw, J., & Graham, M. (2017). An Informational Right to the City? Code, Content, Control, and the Urbanization of Information.
Antipode.
Taylor, L. (2017). What is data justice? The case for connecting digital rights and freedoms globally.
Big Data & Society,
4(2), 2053951717736335.